STRATEGY & INSIGHTS

6 min read
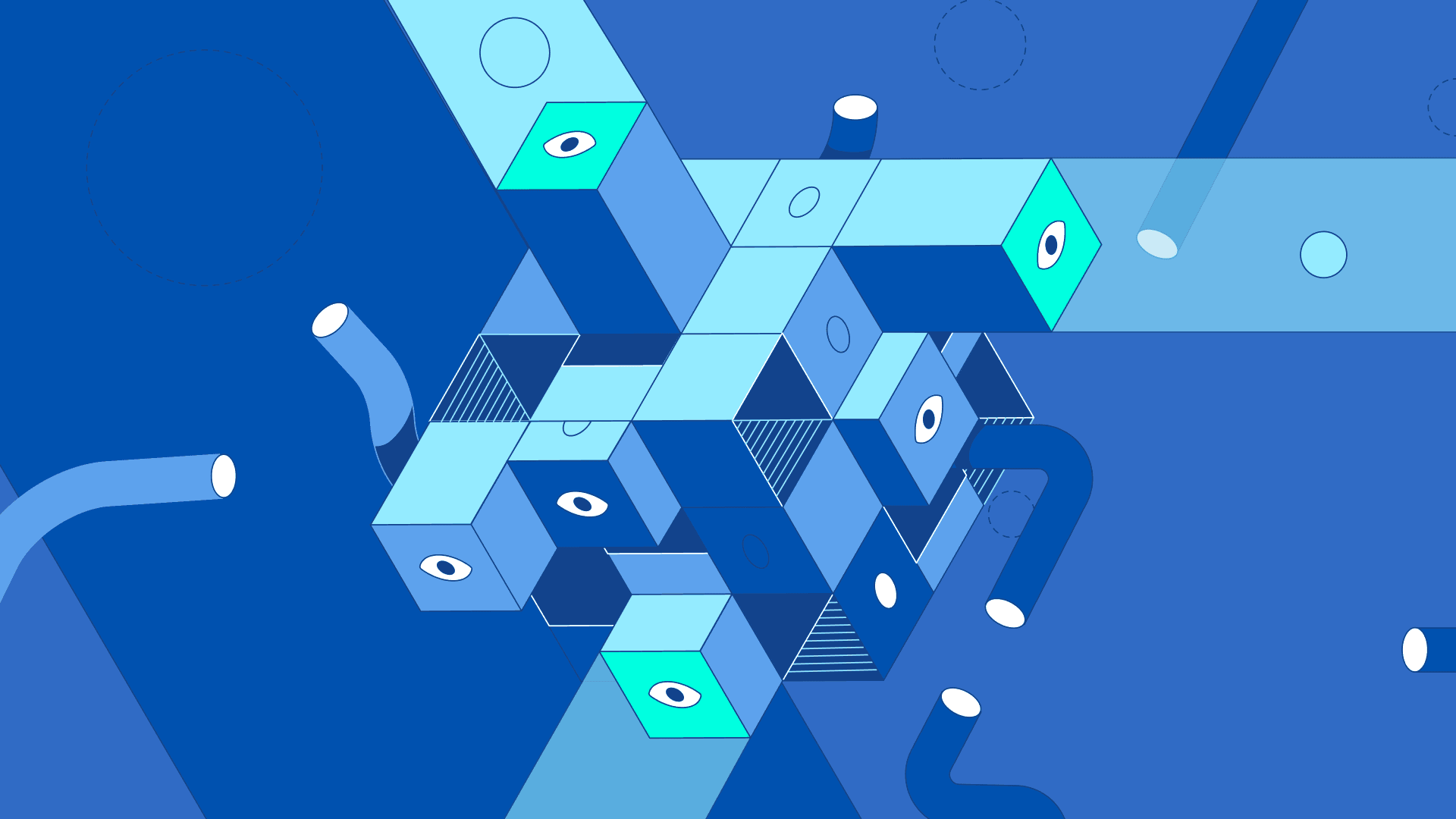

Share
Published on 10/03/2023
Last updated on 02/04/2025
With AI in your enterprise, will users trust you more… or less?
Share
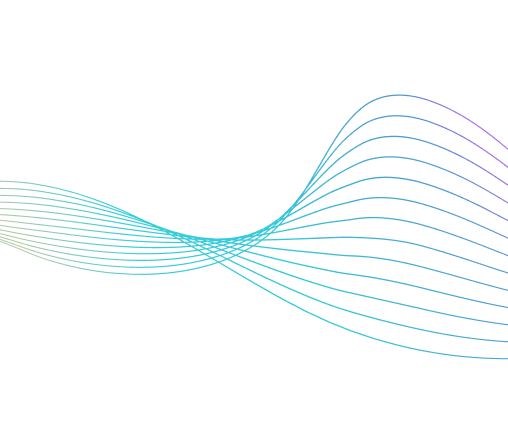
Get emerging insights on emerging technology straight to your inbox.
If predictive AI can make accurate recommendations based on historical data, could you use HR data and KPIs to predict which employees are most likely to underperform next year? Could you use generative AI to provide automated and personalized counseling to people in crisis? Applications like these are certainly possible. But what effect would they have on the trustworthiness of your organization?
The role of AI continues to expand across all sectors, and every enterprise wants to jump on board. But as they look to harness AI’s capabilities, they face an even deeper challenge: building trust from their users. How will they safeguard the data and processes that come with tapping into AI?
In this post, we'll look at how modern enterprises are integrating AI, shedding light on the key processes involved. As we explore, we’ll touch on the security implications of integrating AI—because unless you implement trustworthy AI, you’re creating security problems rather than AI solutions.
Let’s begin by considering examples of AI in action across industries.
Examples of AI in the enterprise
As a real-world example of AI in the enterprise, let’s consider Babble Labs, which was once a standalone venture until Cisco acquired it in 2020. Babble Labs looked at the challenges of delivering clear audio in video conferencing. Amidst background and non-speech noise, how might software improve a participant’s ability to focus on core meeting content?
Traditional noise reduction methods were limited. So, Babble Labs trained neural networks on hundreds of thousands of hours of speech and noise, along with tens of thousands of hours of room acoustics. By leveraging AI/ML, Babble Labs vastly improved speech clarity and noise reduction for Webex Meetings.
This is just one example of the power of AI, but AI’s ever-growing capabilities promise impactful applications across industries. And from one industry to the next, enterprises need to strike a fine balance between innovative applications and engendering trust.
For example, in healthcare, a medical provider can leverage AI to interpret individual patient histories alongside real-time biometrics, crafting personalized and optimal patient care plans. But what about patients who don’t want their data—even if it’s anonymized—to be used to inform the care of other patients? Or worse, what if data isn’t anonymized or stored securely, and it’s vulnerable to a breach of protected health information?
In emergency management, generative AI can simulate disaster scenarios to help cities prepare emergency response plans, project outcomes, and determine the distribution of resources. But what if it’s built on biased models that prioritize certain demographics over others?
In fintech, financial institutions can use predictive analytics, analyzing consumer spending behavior to craft personalized financial guidance or calculate risk when approving loans. How transparent should these institutions be about the role of AI in influencing what could be life-changing decisions for their customers?
The potential of AI in the enterprise—in every sector—is incredible. To better understand the points at which an enterprise must consider the trust issue, let’s turn our attention to the processes that make AI possible.
Key processes in using AI
Integrating AI into your enterprise involves an intricate sequence of processes that transform raw data into actionable insights. It’s not as simple as plugging in a new tool. Key processes include:
- Data collection: Gather massive volumes of data from various sources.
- Data processing: Collected data undergoes various operations (such as sanitization, standardization, deduplication, and vectorization) performed by a set of specialized tools.
- Data storage: Store both the raw and processed data in preparation for model training and/or fine-tuning.
- Model building and training: Create an ML model and train it on the data, iteratively refining its accuracy and efficiency.
- Model fine-tuning: Adapt a general ML model for specific use cases to ensure the highest degree of relevance and accuracy.
- User interaction with AI (more often in the case of generative AI): Users pose questions, seek answers, and engage with the AI-based system in real time.
Adopting these new processes naturally introduces substantial infrastructure demands:
- Optimized switching to ensure the rapid and efficient flow of data, as AI involves the handling of vast amounts of data
- Increased network bandwidth to handle the transfer of large datasets
- Data stream management to support various sources for data collection, ensuring data quality and guarding against data loss
- Robust and scalable platforms to support computational needs as ML models grow in size and sophistication
The opportunities are attractive, and the technology available makes it achievable. But will going down this path cause your users to trust you more… or less? With each step forward—whether it’s adding an AI-related process or AI-necessitated infrastructure—a new security risk emerges. Every step, every tool, and every interaction is a potential vulnerability.
Implications for security
To ensure you’re building trustworthy AI, you must proactively address the following security concerns:
- Collection of sensitive data: When gathering data, you may inadvertently collect, store, and handle sensitive or personal identifiable information (PII).
- Risks of incorporating confidential data or IP: If proprietary or confidential information unintentionally makes its way into your training data, you risk exposing trade secrets.
- Model vulnerabilities: Malicious attackers may target your models by poisoning, tampering, or introducing bias, undermining the trustworthiness of your AI.
- Data storage security concerns: Storing vast amounts of data requires taking strong measures to ensure its protection from both breaches and internal misuse.
- Vulnerabilities in AI tools and processes: As your integration efforts rely on tools—especially third-party or open-source ones—your software supply chain may amass unseen security gaps or flaws.
These represent some of the most pressing security challenges with AI, but the list is by no means exhaustive. Each enterprise's journey with AI integration will present a unique set of security considerations and potential pitfalls. Nonetheless, if your enterprise wants to use AI while garnering customer trust, then you must:
- Protect your data.
- Protect your processes.
- Protect your tools.
Building AI that builds trust
The transformative possibilities of AI for enterprises—across every sector—are undeniable. However, as we integrate these potentially game-changing capabilities, we cannot avoid the mandate to pursue trustworthy AI. Enterprises must pursue the use of AI that is more than just effective, but also secure.
Outshift is at the forefront of navigating this intricate interplay, committed to guiding enterprises through the journey with an unwavering focus on both the innovation and the trustworthiness of AI.
In our follow-up article, we focus more deeply on the security landscape surrounding AI integration. We’ll cover what you need to confidently embrace AI's transformative power while establishing a security posture that builds trust from your customers.

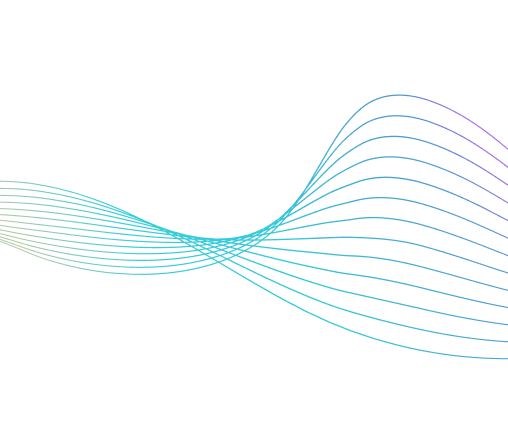
Get emerging insights on emerging technology straight to your inbox.
Welcome to the future of agentic AI: The Internet of Agents
Outshift is leading the way in building an open, interoperable, agent-first, quantum-safe infrastructure for the future of artificial intelligence.
* No email required
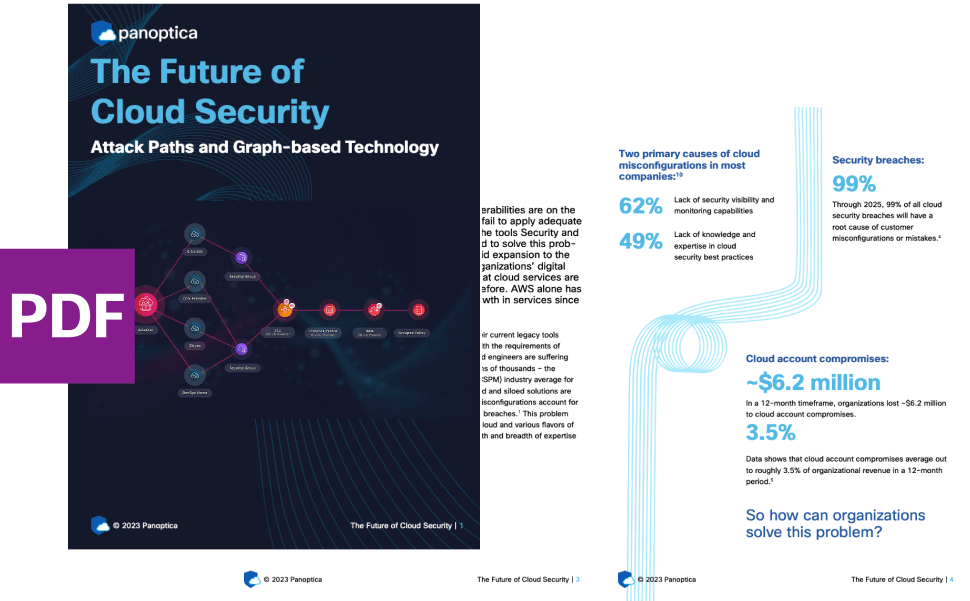
* No email required
Related articles

The Shift is Outshift’s exclusive newsletter.
Get the latest news and updates on generative AI, quantum computing, and other groundbreaking innovations shaping the future of technology.
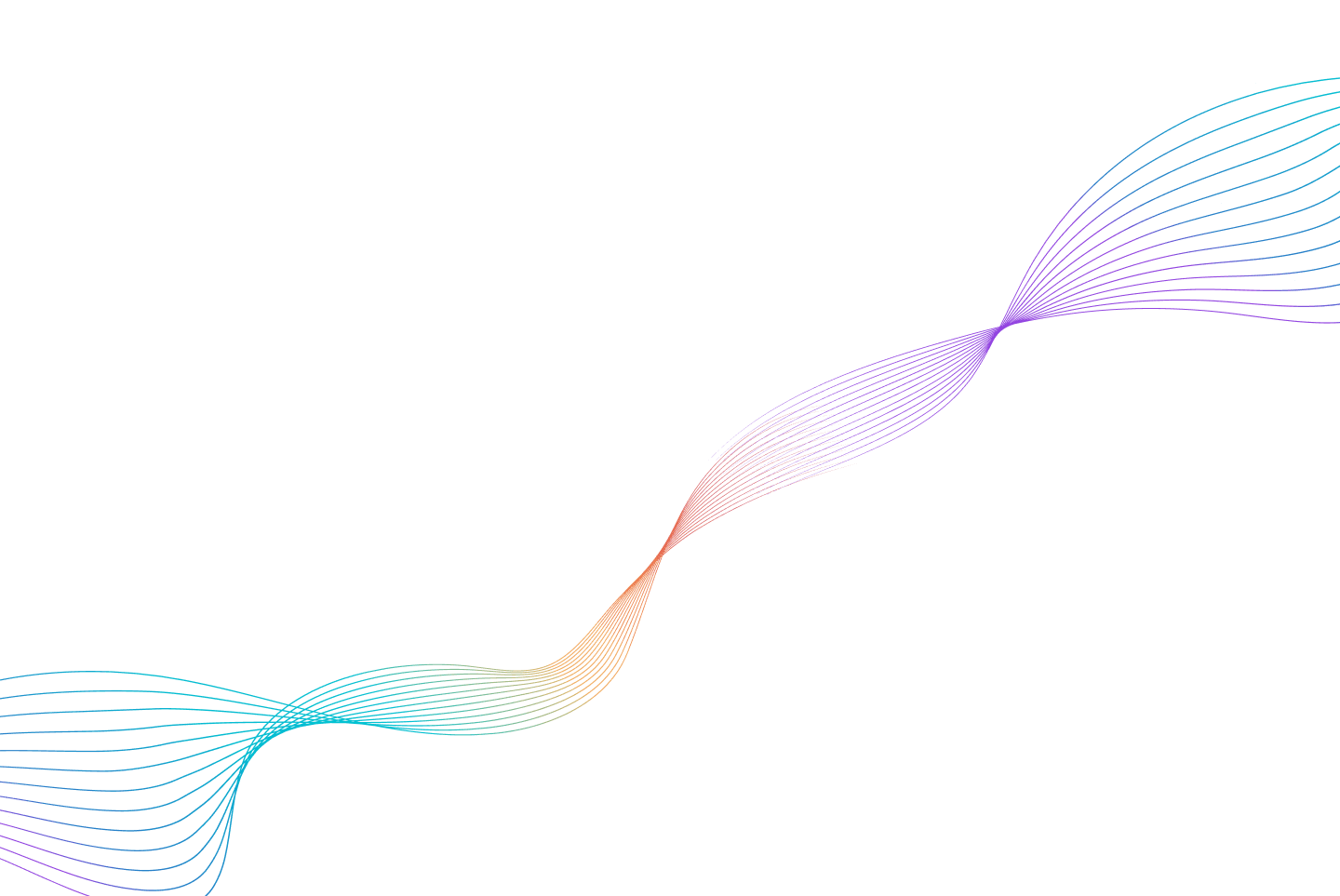